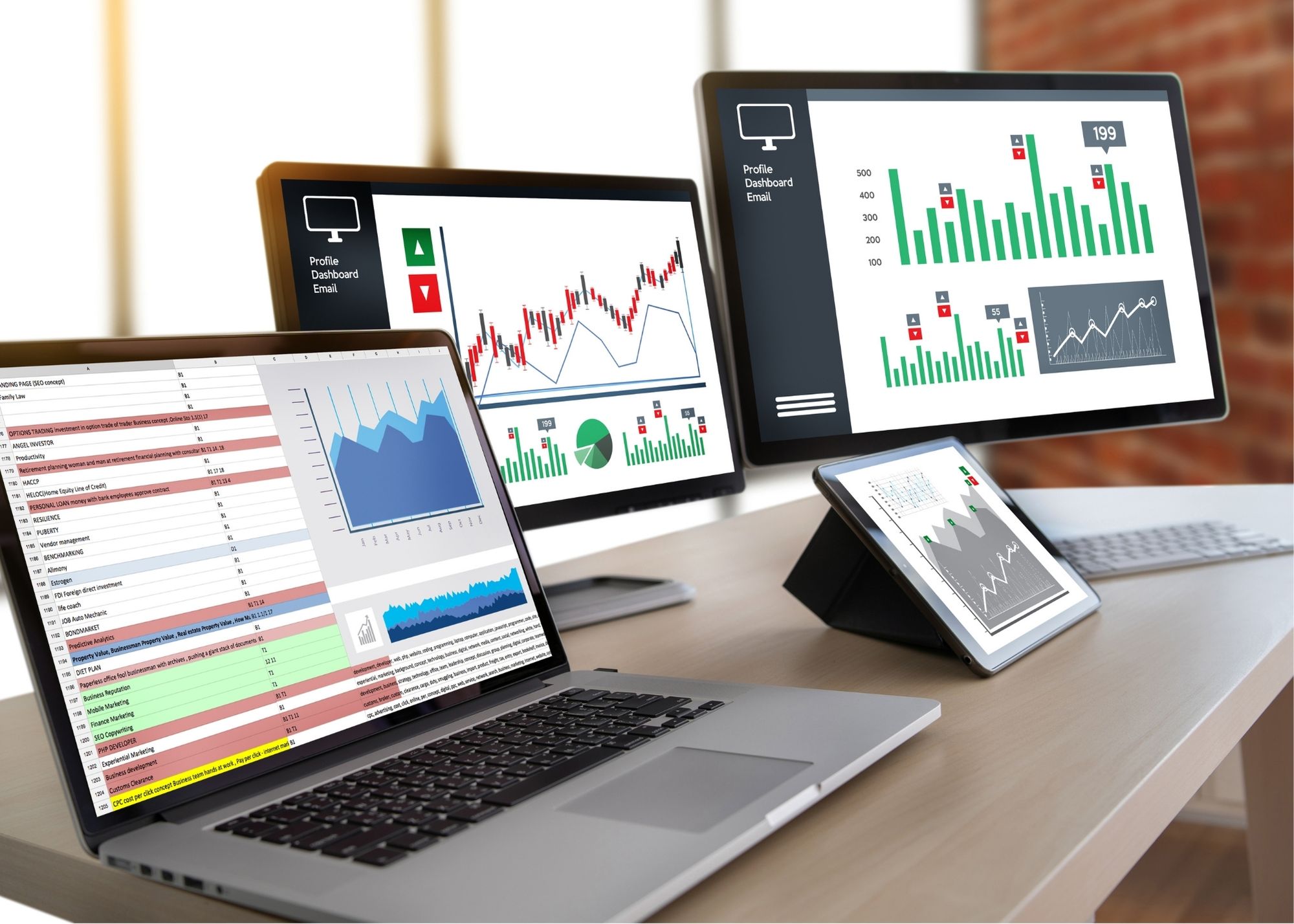
As operational technologies grow with enterprise machine learning and help businesses increase their efficiencies by using data driven solutions to produce specific suggestions and recommendations, so does the impact on IT and data. The use of machine learning on physical assets – like the machinery, tools and sensors used in a production environment – has increased our need to consume the data produced and use it in a practical way to streamline business efforts. Prior to the existence of the cloud – a way for us to store and compute endless information – and the prevalence of machine learning, OT data was typically reviewed and analyzed within the historian program and then selected aggregates of the information, such as total volumes sold or processed, were then distributed down the data “food chain”. This is because the cloud was expensive and less commonly used.
Now, as the cost of using the cloud decreases, we see many organizations focusing on moving their OT data into the cloud. This change has democratized the data and has enabled data engineers and data scientists to experiment with the data, develop models, test them, vet them and ultimately retain the models that add business value. Frequently, the results of the models are looped back into the Supervisory control and data acquisition (SCADA) system or a data historian where they are consumed by an organization’s operations team and used to create everyday efficiencies and contribute their bottom line. Alternatively, output from the cloud in the form of a dashboard or report is then also consumable by the operations team. In the same cloud architecture, that data can be used for business analytics and reporting – ultimately, driving business productivity.
We now know that machine learning has helped further converge the gap between IT and OT. Frequently, enterprise machine learning results have flowed back into OT systems. This enables the machine learning recommendations to be made in the same platform the operators see of the other data. Not only does the data now have to flow from the OT to IT world reliably, but it also must go back reliably. This tighter coupling has led many of Arcurve’s clients prioritizing how operational data flows within the organization. A greater OT data landscape allows your business to understand it’s assets at a more granular level. This strategy is foundational to advancing analytics and AI in your business.
This is part two in a blog series on Operational Technologies and Machine Learning. Stay tuned as we dig deeper into how OTs can help your business.
If you’re interested in reading more about how Operational Technologies, check out our informative four-pager here. If you’d like to chat about how Arcurve’s Advanced Analytics team can help you use OT to drive efficiencies in your business, reach out to us below.