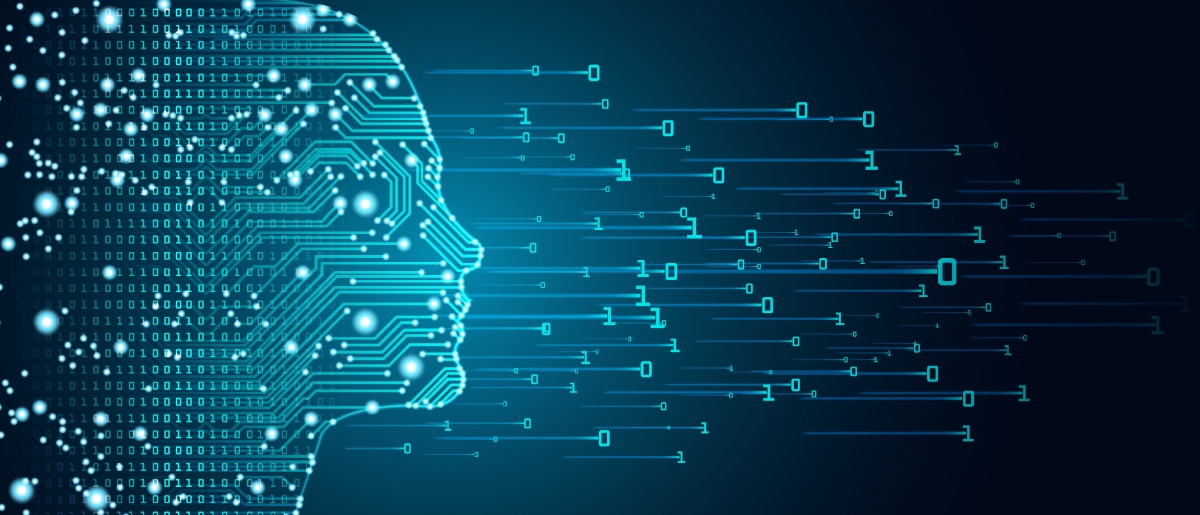
Our clients are always looking for ways to optimize their business and uncover new revenue streams. The realm of data science today is particularly relevant because many of our clients have data sets that were collected, in some cases over decades, for a specific purpose but undoubtedly have latent or extended value just waiting to be uncovered. In other cases, our clients have domain-specific expertise that can be used to unlock value in public data sets or those owned by other entities.
We’ve completed a wide range of Data Visualization and Data Analytics projects which are important and necessary precursors to Machine Learning, Big Data, and Artificial Intelligence. Where Data Analytics looks to historical data to understand “what happened," the goal of Machine Learning is to be predictive. Examples include the identification of non-obvious opportunities, prediction of impending failure, detection of anomalies, or optimization of a dynamic process.
The key to Machine Learning is that a mathematical model of the domain problem needs to be developed, which must be sufficiently sophisticated to process input data and reliably and accurately make the prediction. The model is trained and verified against historical data with known outcomes. New data is then fed through the trained model to forecast the predictions.
When Arcurve embarks on a Machine Learning project, the approach we take tends to follow a pattern. First, we work with the client to understand the data available and the prediction to be made. We then work with the client’s domain experts to develop an appropriate mathematical model that is representative of the domain problem. The model is trained with known data and outcomes, the results are evaluated, the model is refined, and the process is repeated until an acceptable level of accuracy is achieved. In many situations, especially with high-frequency real-time data, we see that new “edge cases” are uncovered over time. These result in new learnings which are fed back into the model and training process and illustrate that effective use of Machine Learning is a journey.
When you are ready to begin your journey into the world of Machine Learning, give us a call.